How Machine Learning is Transforming Healthcare: Innovations and Challenges
7 min read
14 Aug 2024
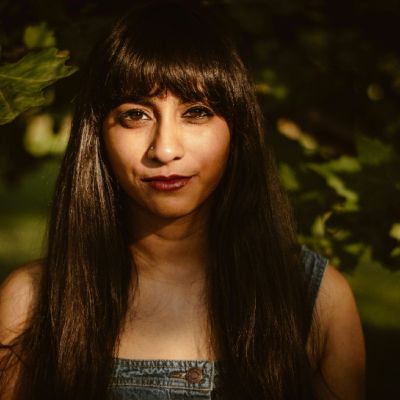
Machine learning (ML), a subset of artificial intelligence, is revolutionizing the healthcare industry by providing innovative solutions to complex problems. From early disease detection to personalized treatment plans, ML is reshaping the landscape of healthcare. This article explores the key innovations driven by machine learning in healthcare and the challenges that need to be addressed for its broader adoption.
Early Disease Detection and Diagnosis
One of the most significant impacts of machine learning in healthcare is its ability to detect diseases early and accurately. ML algorithms analyze vast amounts of medical data, including imaging, genetic information, and patient histories, to identify patterns and markers associated with specific conditions. For instance, in radiology, ML models can assist radiologists in identifying abnormalities in medical images such as X-rays, MRIs, and CT scans. Google's DeepMind has developed an algorithm that can detect over 50 eye diseases with high accuracy, often matching or exceeding the performance of human specialists.
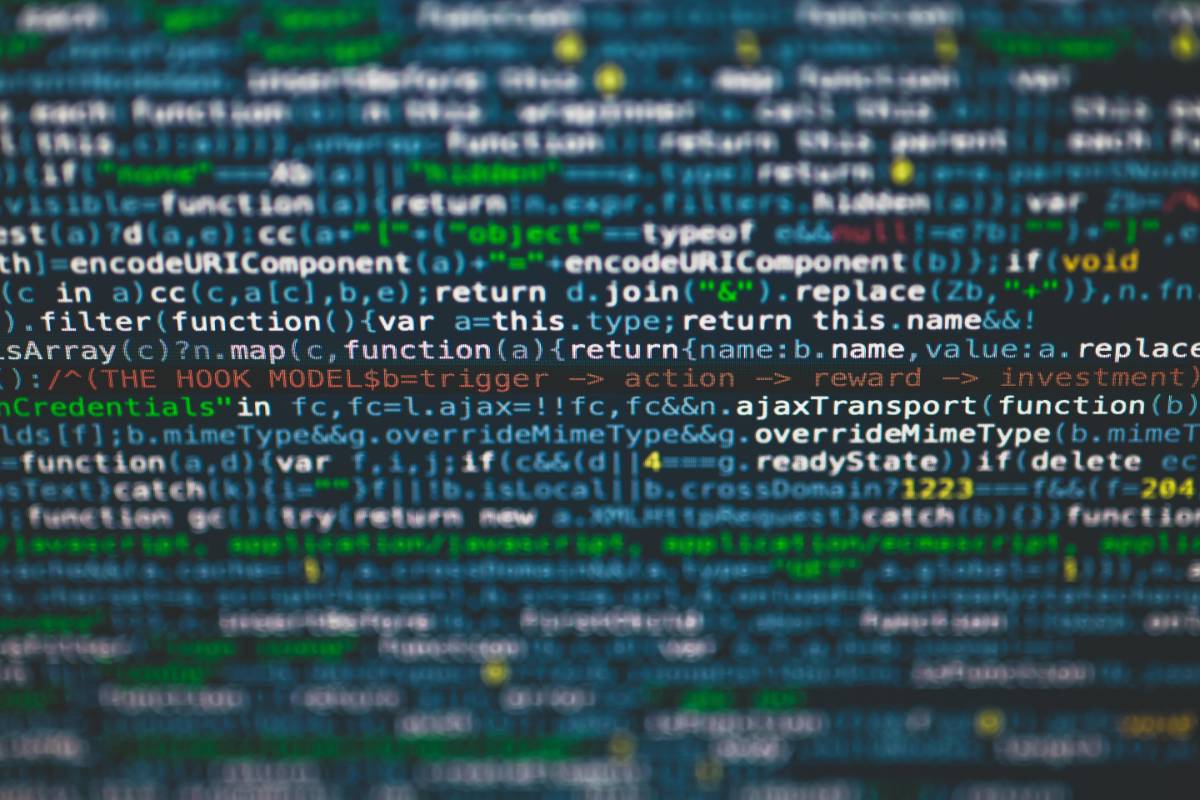
Personalized Treatment Plans
Machine learning is also pivotal in developing personalized treatment plans tailored to individual patients. By analyzing data from various sources, including electronic health records (EHRs), genetic profiles, and lifestyle information, ML models can predict how patients will respond to different treatments. This personalized approach is particularly beneficial in oncology, where treatment responses can vary significantly between patients. For example, IBM Watson for Oncology uses machine learning to provide oncologists with evidence-based treatment options based on the patient’s unique medical data.
Predictive Analytics and Patient Monitoring
Predictive analytics powered by machine learning is transforming patient monitoring and management. Hospitals are using ML models to predict patient deterioration, readmission rates, and the likelihood of complications. This allows healthcare providers to intervene proactively, improving patient outcomes and reducing hospital stays. Wearable devices and remote monitoring systems, equipped with ML algorithms, continuously analyze patient data to detect early signs of health issues. This real-time monitoring is crucial for managing chronic diseases such as diabetes, where timely interventions can prevent severe complications.
Drug Discovery and Development
The drug discovery and development process is notoriously time-consuming and expensive. Machine learning is accelerating this process by identifying potential drug candidates more efficiently. ML algorithms analyze biological data to uncover new drug targets and predict the efficacy and safety of drug compounds. For instance, companies like BenevolentAI and Atomwise use machine learning to sift through vast datasets, significantly reducing the time and cost associated with traditional drug discovery methods. Additionally, ML is helping to repurpose existing drugs for new therapeutic uses, offering new hope for diseases with limited treatment options.
Administrative Efficiency
Beyond direct patient care, machine learning is enhancing administrative efficiency in healthcare settings. ML models streamline administrative tasks such as scheduling, billing, and resource allocation, reducing operational costs and minimizing human error. Natural language processing (NLP), a branch of machine learning, is being used to automate the extraction of relevant information from clinical notes, improving the accuracy of patient records and freeing up healthcare professionals to focus more on patient care.
Challenges and Ethical Considerations
Despite its transformative potential, the integration of machine learning in healthcare faces several challenges. Data privacy and security are paramount concerns, as ML models require access to sensitive patient data. Ensuring that this data is anonymized and securely stored is crucial to maintaining patient trust. Additionally, the quality and diversity of the data used to train ML models can impact their accuracy and fairness. Biases in the data can lead to disparities in healthcare outcomes, particularly for underrepresented populations. Addressing these biases and ensuring equitable access to ML-driven healthcare solutions is essential.
Another challenge is the interpretability of ML models. Many ML algorithms, particularly deep learning models, operate as "black boxes," making it difficult to understand how they arrive at specific predictions. This lack of transparency can hinder the adoption of ML in clinical settings, where explainability is crucial for gaining the trust of healthcare providers and patients.
The Future of Machine Learning in Healthcare
Looking ahead, the future of machine learning in healthcare is promising. Advances in AI and ML technologies, coupled with increasing amounts of healthcare data, will continue to drive innovation. Collaborative efforts between technology companies, healthcare providers, and regulatory bodies are essential to overcome current challenges and unlock the full potential of machine learning in healthcare.
In conclusion, machine learning is transforming healthcare by enabling early disease detection, personalized treatments, and efficient patient monitoring. While challenges remain, the ongoing advancements in ML technology and a commitment to ethical practices will pave the way for a future where healthcare is more precise, efficient, and accessible.
More Articles
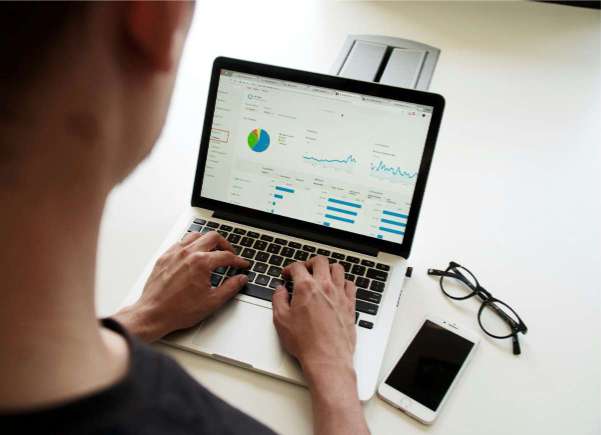
The Secret to Effective Data Governance Revealed!
4 min read | 17 Jul 2024
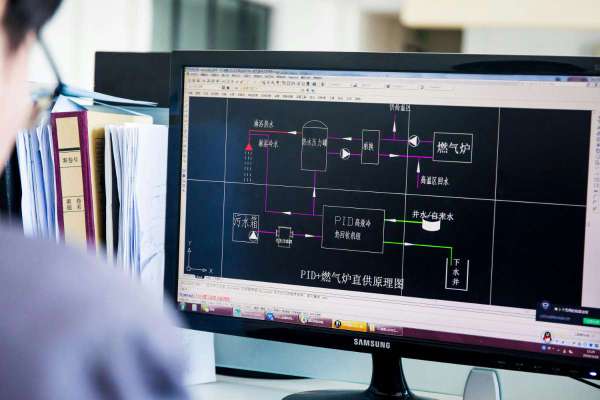
Unlock the Power of Business Intelligence (BI) – The Ultimate Guide
7 min read | 16 Jul 2024
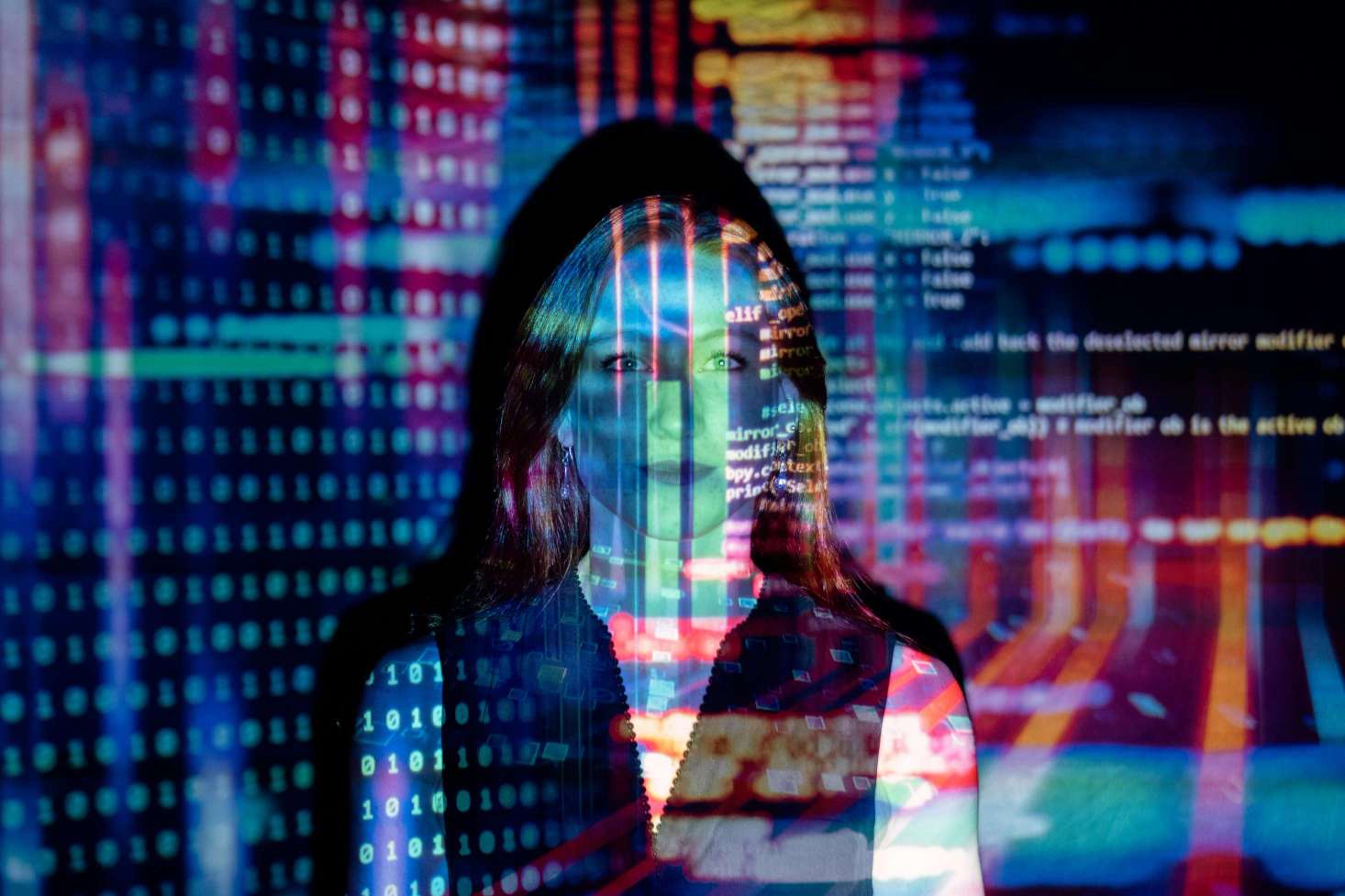
Data Lakes vs. Data Warehouses: Which One Do You Really Need?
6 min read | 15 Jul 2024
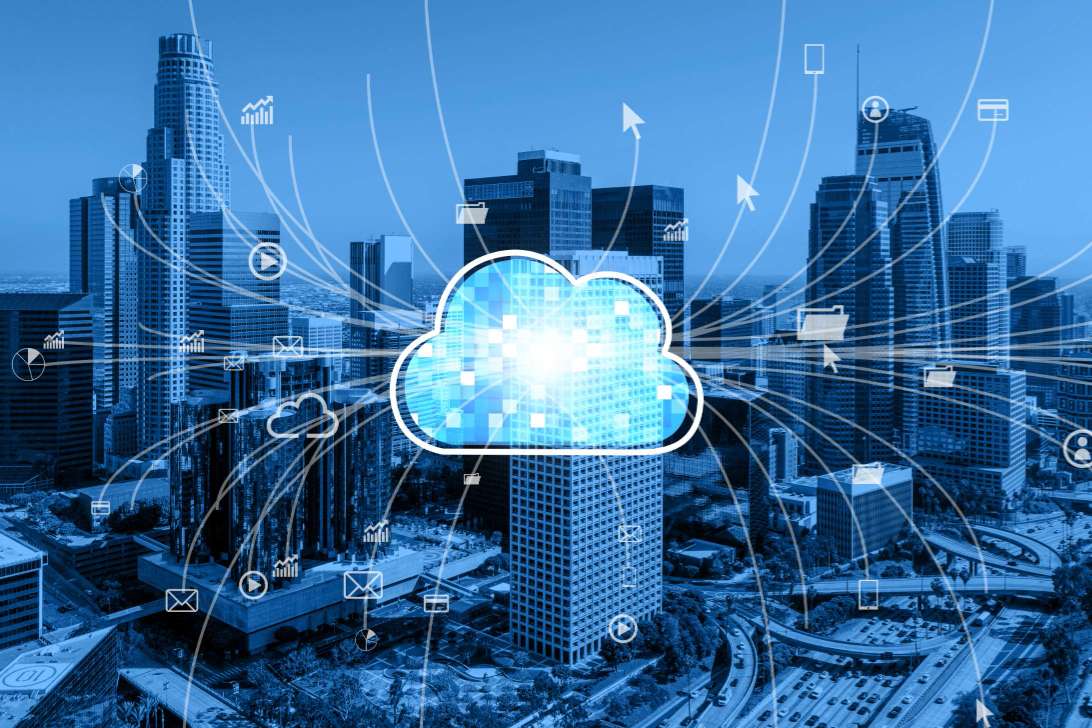
10 Incredible Benefits of Cloud Storage You Didn't Know About
6 min read | 14 Jul 2024
More Articles
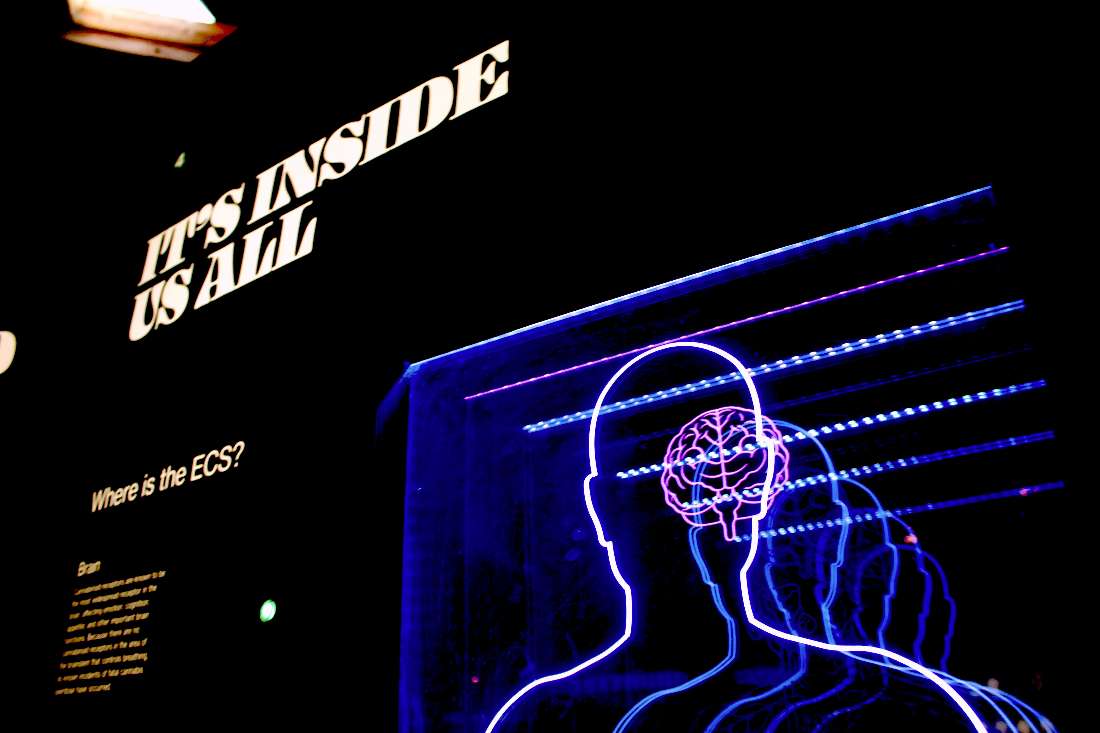
Brain-Computer Interfaces: The Frontier of Human-Computer Interaction
6 min read | 07 Sep 2024
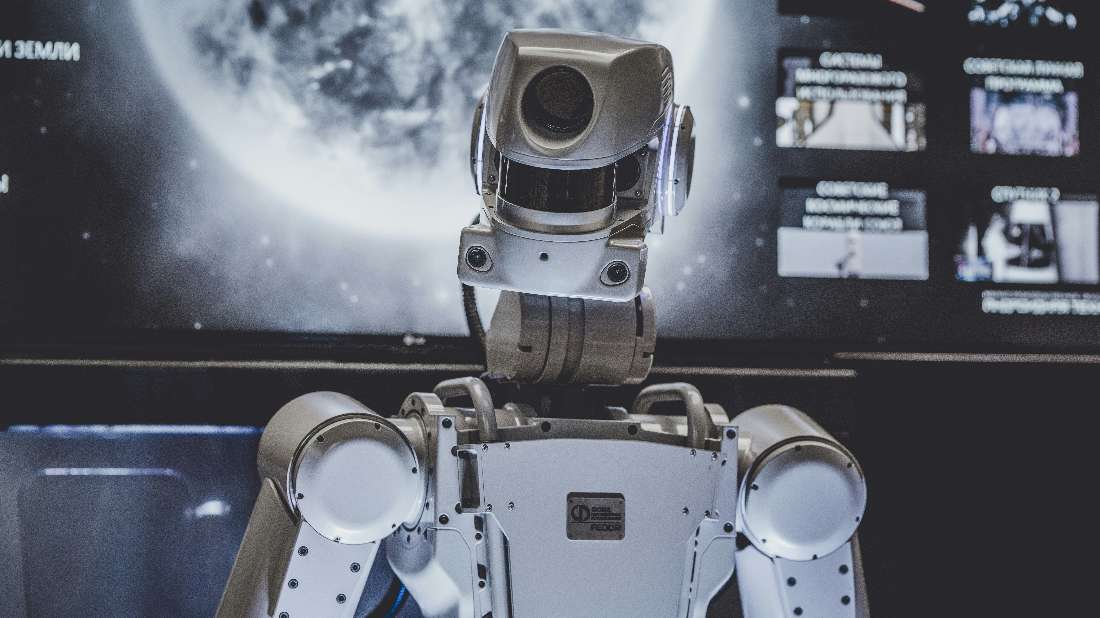
AI Ethics and Bias: Ensuring Fairness in Machine Learning
7 min read | 06 Sep 2024
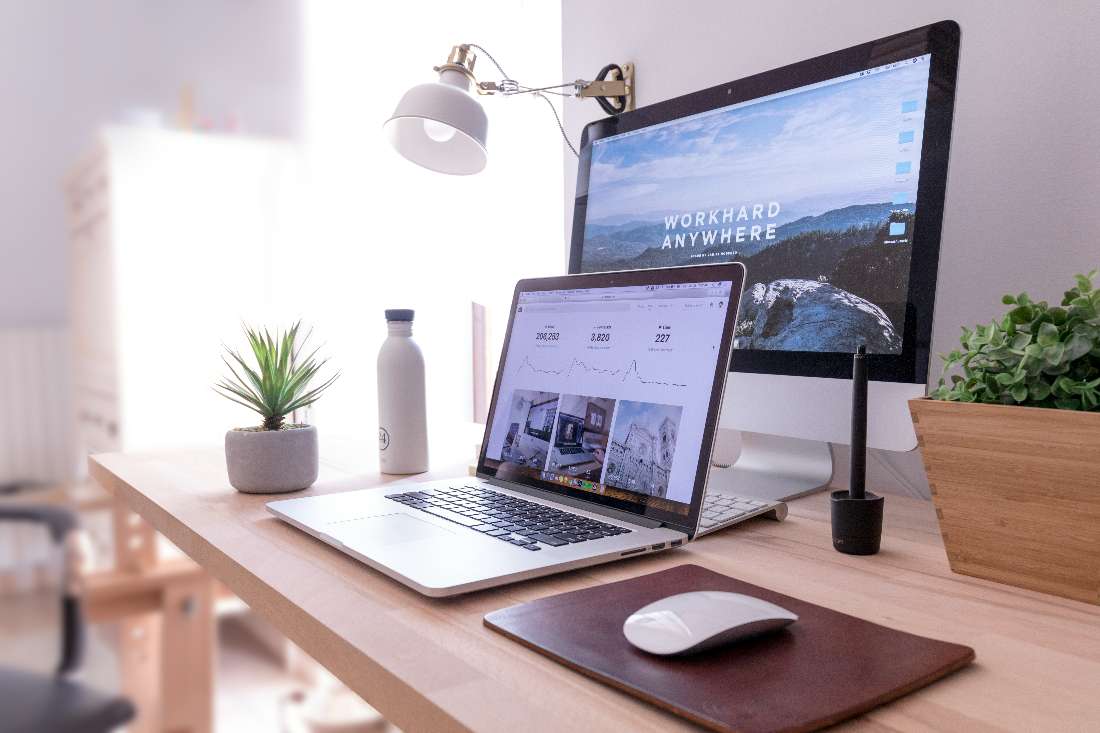
Edge Computing: Decentralizing Data Processing
7 min read | 05 Sep 2024
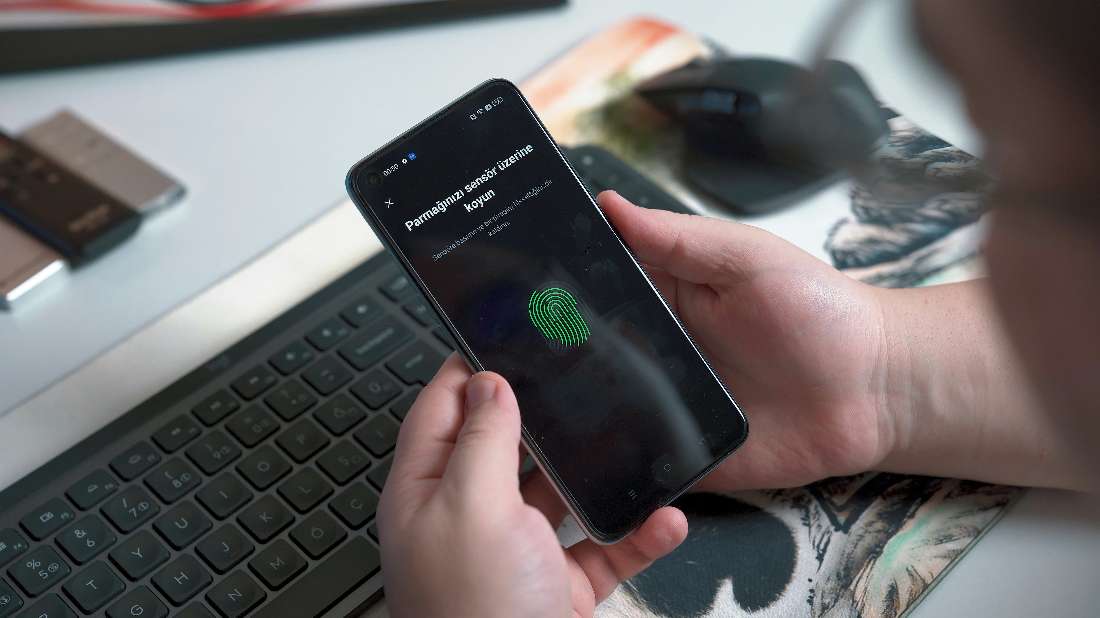
The Future of Biometric Security: Beyond Passwords
7 min read | 04 Sep 2024