Bias in AI: Addressing Fairness and Inclusivity in Machine Learning Models
5 min read
02 Sep 2024
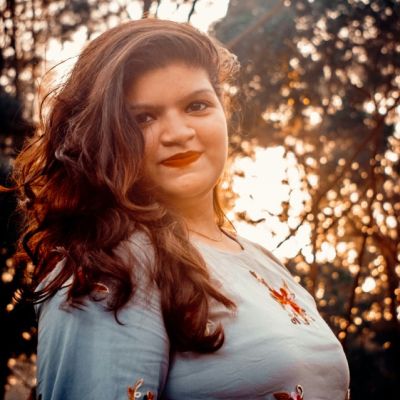
Bias in artificial intelligence (AI) models poses significant challenges to fairness, accountability, and inclusivity across various applications. This article explores the complexities of bias in AI, its impact on decision-making processes, and strategies to promote fairness and inclusivity in machine learning systems.
Understanding Bias in AI
Bias in AI refers to systematic errors or unfairness in machine learning models that lead to discriminatory outcomes against certain individuals or groups. Bias can arise from biased training data, flawed algorithms, or inadequate consideration of societal biases embedded in data collection processes. Types of bias include algorithmic bias, dataset bias, and interaction bias, which affect model predictions and decision-making processes in areas such as hiring, lending, and criminal justice.
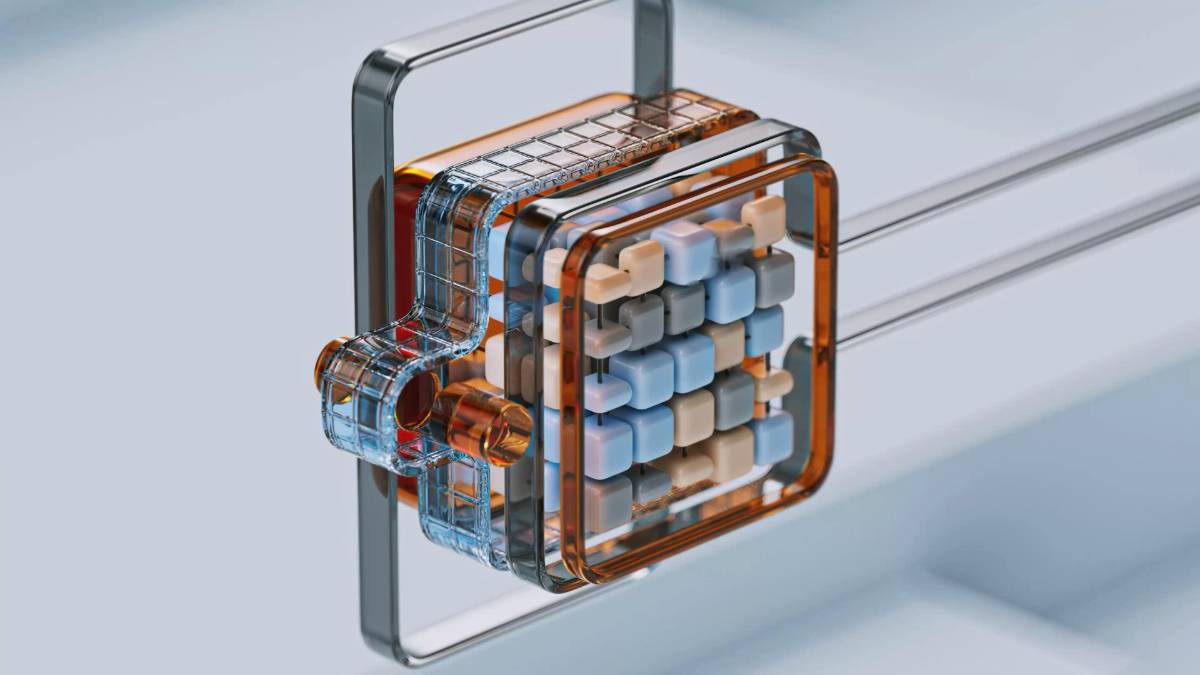
Impact on Fairness and Accountability
Biased AI models perpetuate and amplify societal inequalities by making decisions that disadvantage marginalized groups or reinforce existing biases. In sectors like finance, healthcare, and law enforcement, biased algorithms can lead to unfair treatment, unequal opportunities, and reduced trust in AI-driven systems. Addressing bias is crucial for ensuring fairness, accountability, and transparency in AI applications that impact individuals' lives and societal outcomes.
Strategies for Mitigating Bias
Mitigating bias in AI requires a multi-faceted approach that includes diverse representation in AI development teams, rigorous data preprocessing to detect and mitigate biases in training data, and implementing fairness-aware algorithms. Techniques such as bias detection metrics, fairness constraints, and adversarial testing help identify and mitigate biases throughout the AI development lifecycle. Ethical guidelines and regulatory frameworks also play a vital role in promoting responsible AI deployment and mitigating unintended consequences.
Promoting Inclusivity and Diversity
Promoting inclusivity in AI involves ensuring diverse representation in dataset collection, algorithm design, and decision-making processes. Engaging diverse stakeholders and community feedback in AI development fosters awareness of biases and promotes inclusive practices that prioritize equitable outcomes. Collaboration between AI researchers, policymakers, and affected communities is essential to address bias, uphold human rights principles, and build AI systems that serve diverse societal needs effectively.
Challenges and Ethical Considerations
Addressing bias in AI involves challenges such as defining fairness metrics, balancing trade-offs between accuracy and fairness, and navigating ethical dilemmas in decision-making. Ethical considerations include transparency in AI decision-making processes, accountability for AI outcomes, and safeguarding privacy and human rights in data-driven environments. Continued research, education, and collaboration are essential to develop AI technologies that align with ethical principles and societal values.
Future Directions
The future of addressing bias in AI lies in advancing research in fair AI algorithms, integrating diverse perspectives in AI development, and enhancing regulatory frameworks for ethical AI deployment. Innovations in interpretability and explainability of AI models aim to increase transparency and accountability in decision-making processes. By prioritizing fairness, inclusivity, and ethical considerations, stakeholders can mitigate bias and foster trust in AI technologies as tools for positive societal impact.
In conclusion, addressing bias in AI is critical for promoting fairness, accountability, and inclusivity in machine learning models across various sectors. By implementing strategies to mitigate bias, promoting diversity in AI development, and upholding ethical standards, stakeholders can ensure that AI technologies contribute to equitable outcomes and societal well-being in the digital age.
More Articles
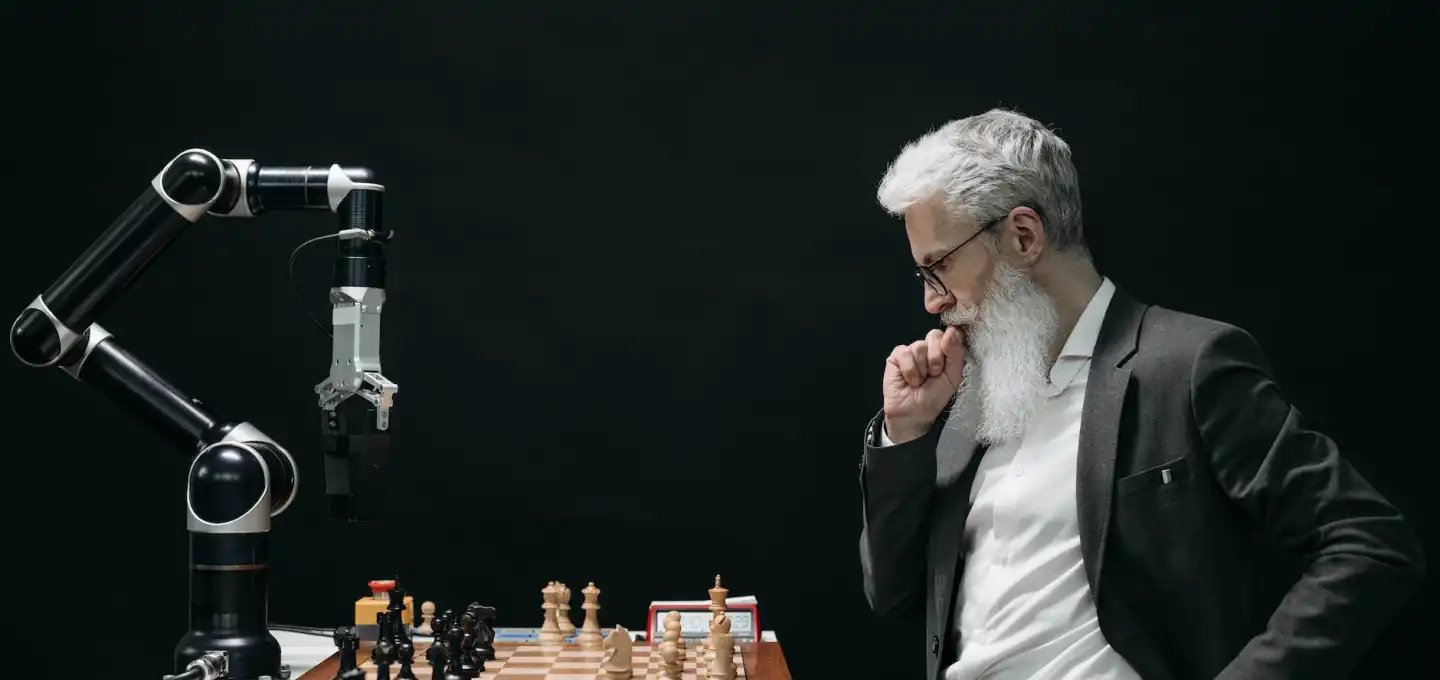
Mind-Reading Technology: The Future of Brain-Computer Interfaces
5 min read | 16 Apr 2024
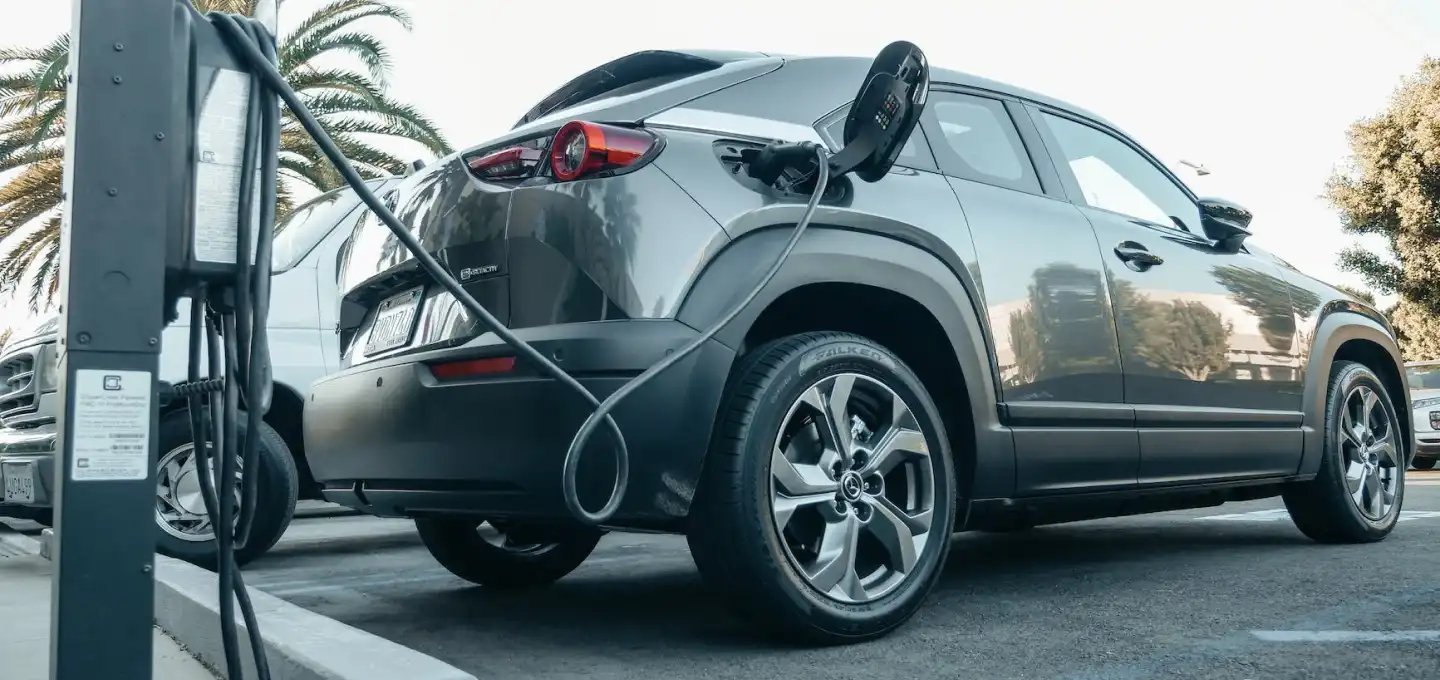
Electric Vehicles: Revolutionizing the Automotive Industry
2 min read | 15 Apr 2024
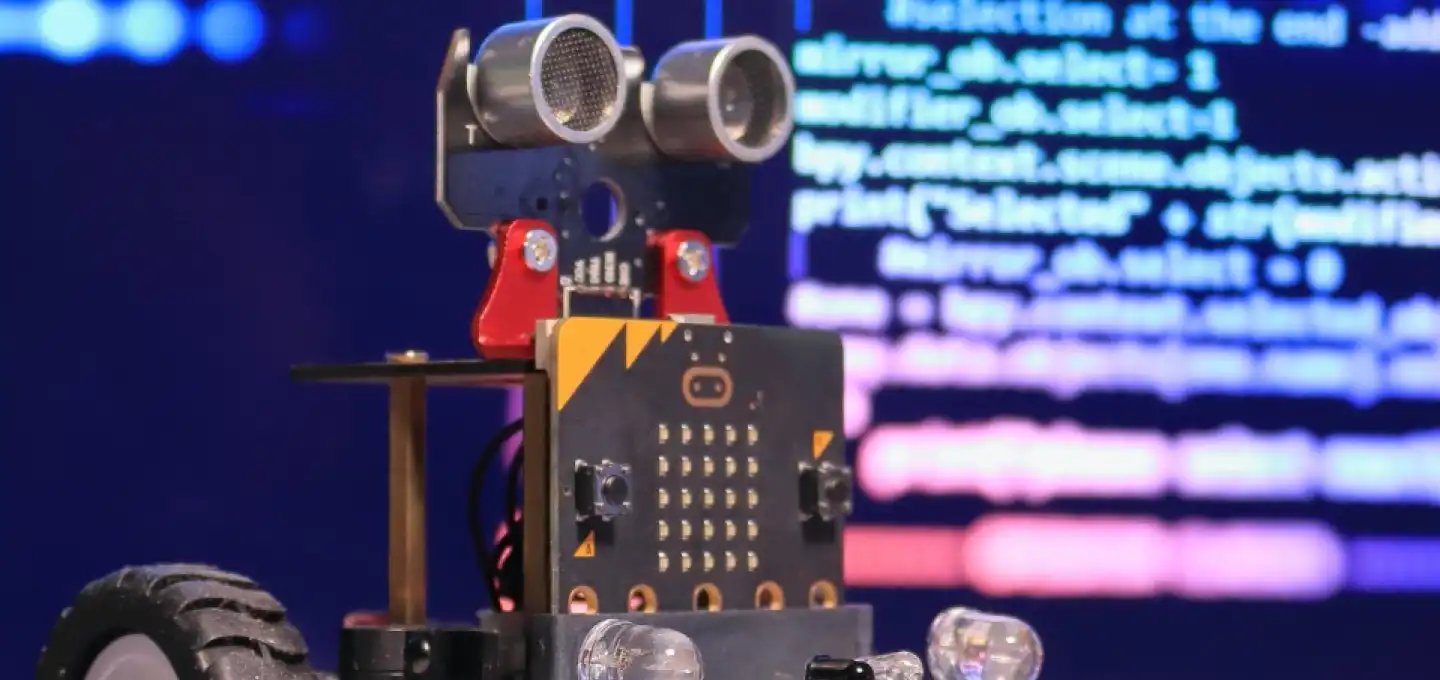
Artificial Intelligence in Education: Personalized Learning and Beyond
4 min read | 14 Apr 2024

Robots in Everyday Life: From Companion Bots to Delivery Drones
3 min read | 13 Apr 2024
More Articles
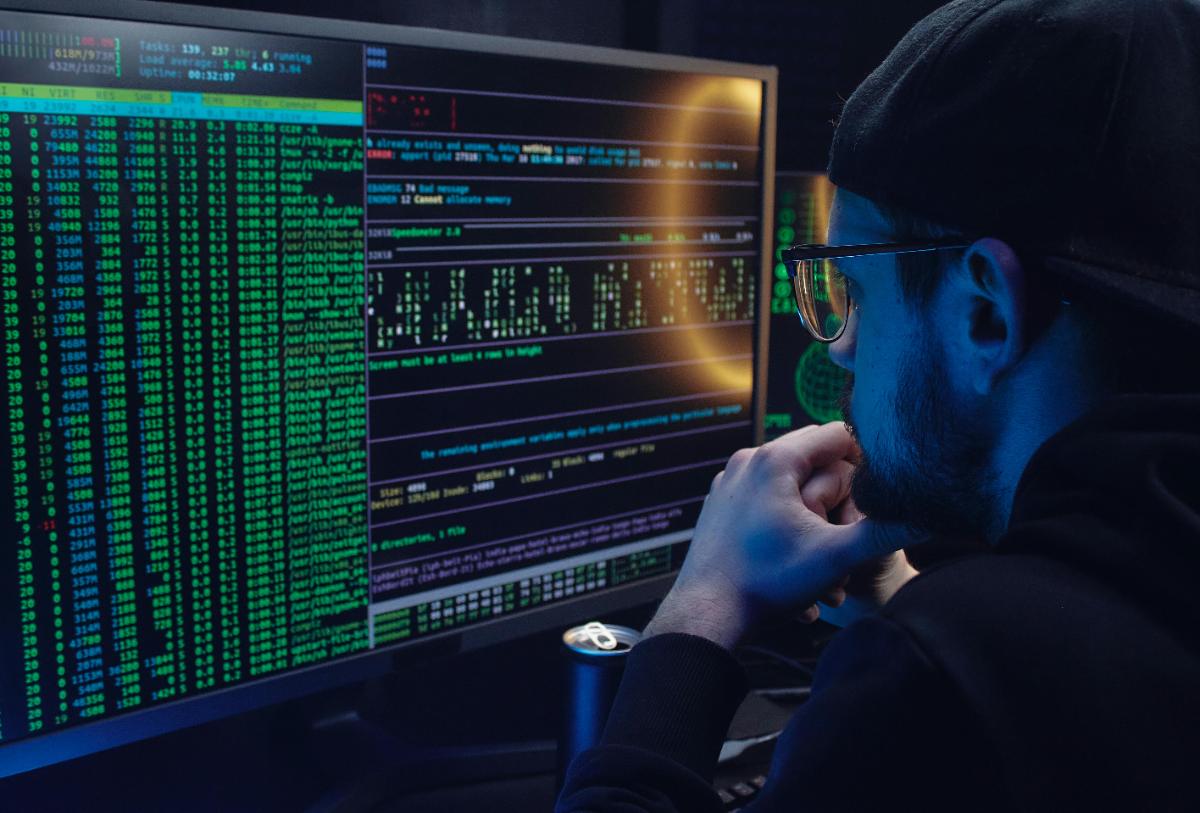
Hacking the System: Ethical Hacking for a More Secure Blockchain
6 min read | 08 Sep 2024
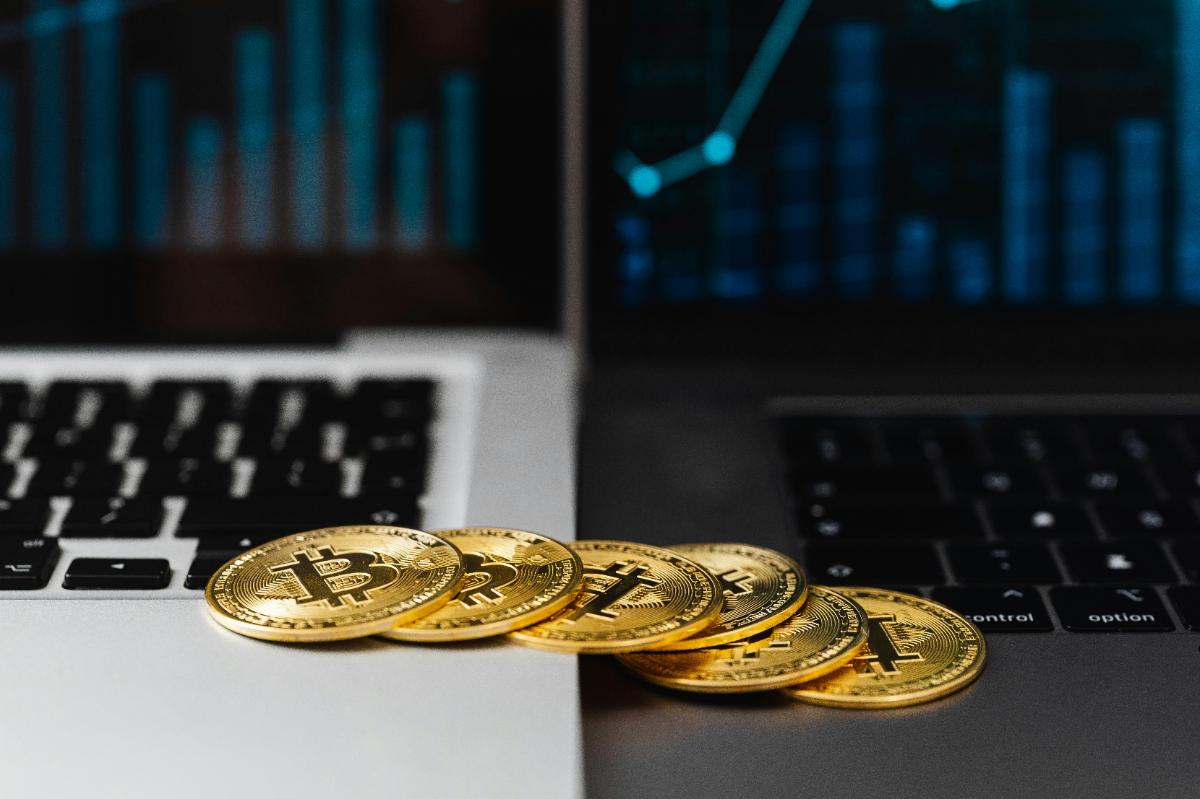
The Energy Paradox: Powering the Blockchain Revolution Sustainably
6 min read | 07 Sep 2024
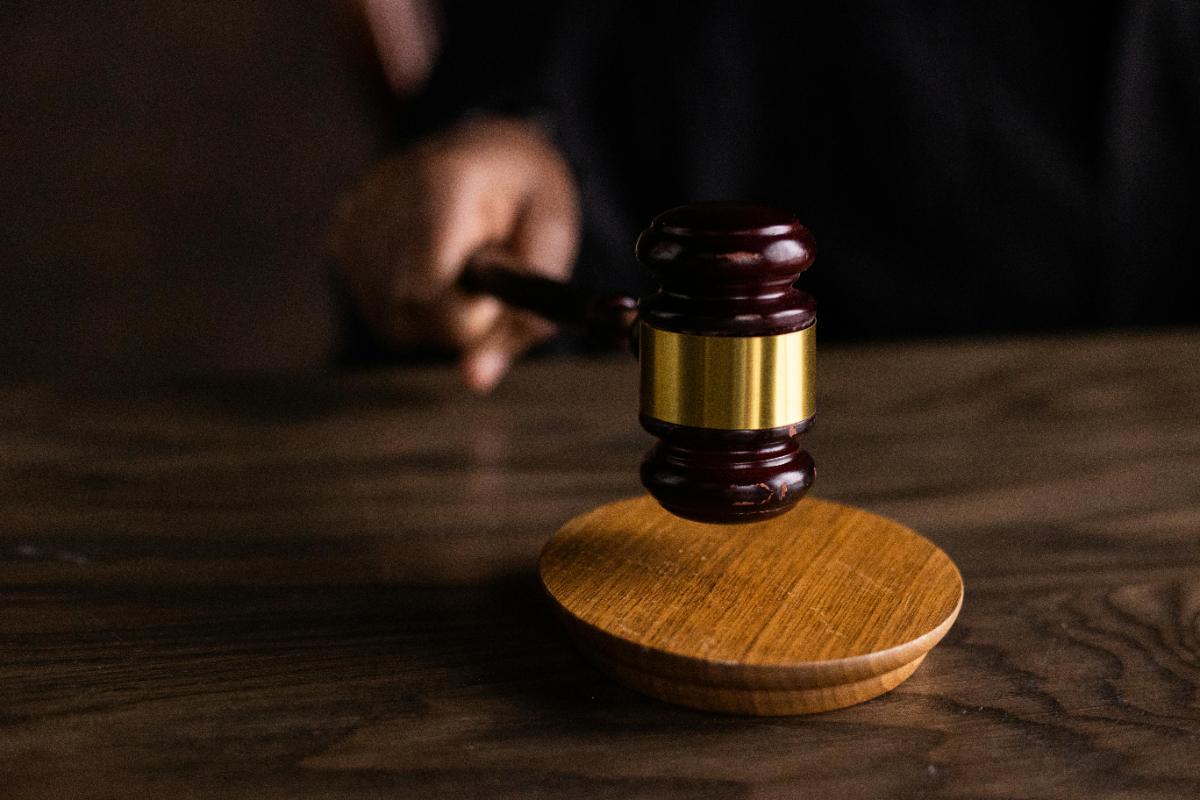
Decentralized Justice: Can Blockchain Revolutionize Law Enforcement?
4 min read | 06 Sep 2024
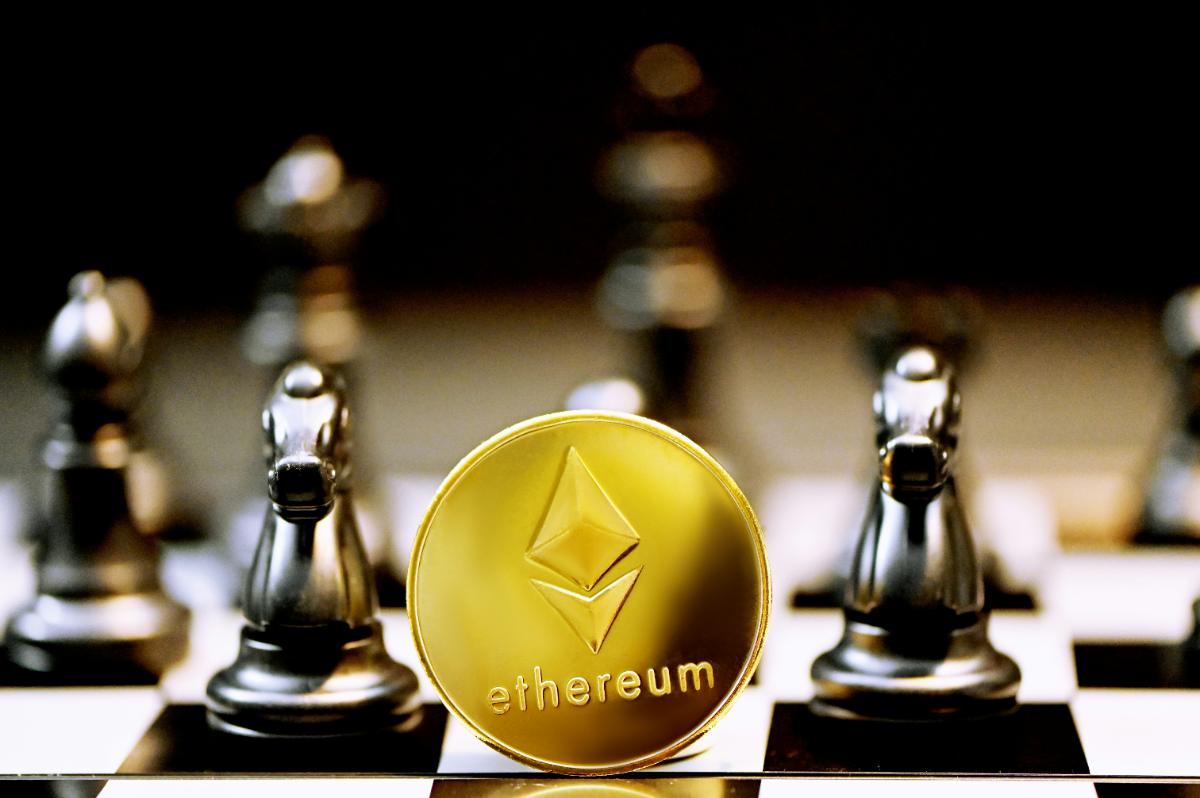
Beyond Crypto-Kitties: The Future of Blockchain Gaming
6 min read | 05 Sep 2024